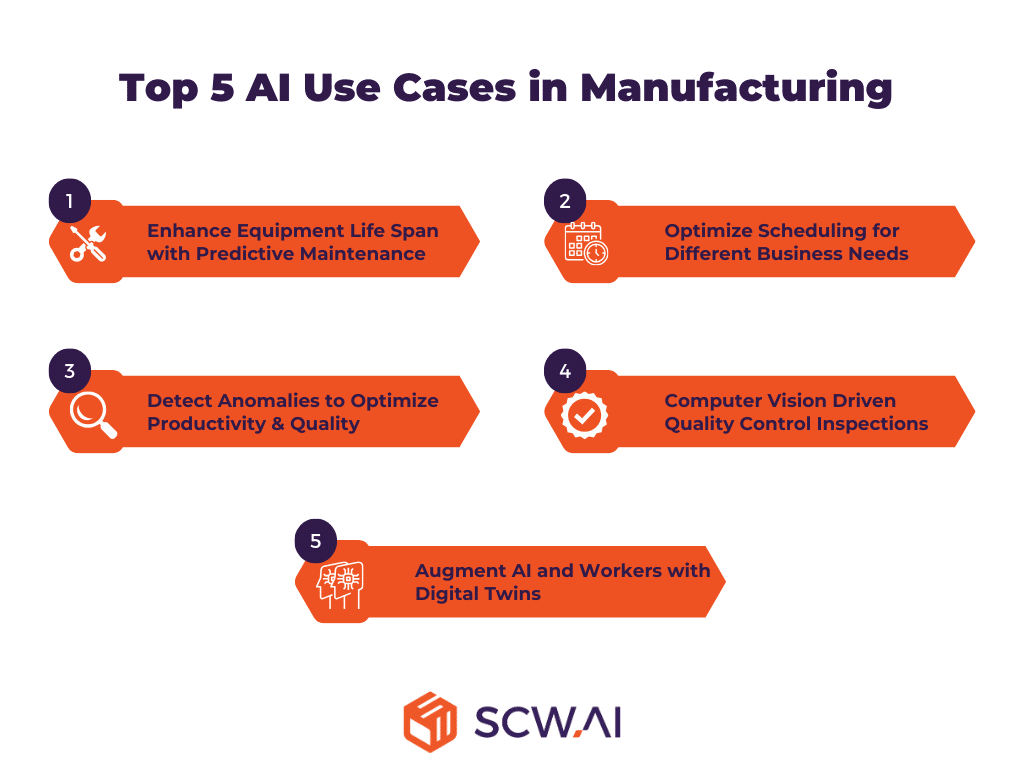
Although manufacturers are aware of how AI can improve and automate decision-making, a recent EY survey has revealed a notable absence of a structured strategy for implementing AI in the manufacturing industry. Only 10% of manufacturers have a clear plan in place for utilizing AI to improve their manufacturing capabilities. This shortcoming might result from a lack of knowledge about the AI use cases in the manufacturing industry.
In an effort to enlighten manufacturing leaders about the practical applications of AI in the shop floor, we aim to spotlight five distinct use cases of AI/ML models tailored specifically to the manufacturing industry. It is important to note that in this article, we intentionally exclude generative AI use cases or generic AI applications applicable to any industry, such as the automation of document processes or the enhancement of design creativity. After introducing AI use cases in manufacturing, we will address frequently asked questions concerning AI in manufacturing and illustrate how SCW.AI supports manufacturers in harnessing AI to generate business value.
1. Enhance Equipment Life Span with Predictive Maintenance
Algorithms can predict equipment breakdowns before they occur and notify workers to take preventive actions (see figure below). This enables manufacturers to optimize maintenance activities, minimizing downtime up to 20% and reducing maintenance costs up to 10% per Deloitte.
The cost reduction achieved by AI/ML models in maintenance activities stems from two key dimensions. Firstly, these models automate the decision-making processes of the maintenance scheduling team, particularly in determining schedules for predictive/preventive maintenance activities. As a result, the scheduling team can save up to 50% of the time previously allocated for creating such schedules, allowing them to redirect their efforts toward other scheduling activities.
Secondly, in the absence of AI algorithms, the maintenance team is faced with a choice between reactive maintenance, initiated when the equipment stops working, and preventive maintenance based on the team’s experiential knowledge, which is often prone to errors. Within this dichotomy, overall equipment effectiveness (OEE) tends to remain low due to unplanned downtimes or unwarranted planned maintenance activities. Predictive maintenance seeks to strike a balance by leveraging historical shop floor data to minimize planned maintenance activities in the most effective manner.
To find out more information regarding predictive maintenance with AI/ML models, you can read our Predictive Maintenance with Machine Learning article.
2. Optimize Scheduling for Different Business Needs
Thanks to AI capabilities, advanced planning and scheduling systems distribute work orders to production lines effectively based on the selected objective criterion such as:
- Cost minimization
- OTIF maximization
- Makespan minimization
- Just in time production (JIT) and more.
AI schedulers are a critical investment focus for manufacturers, as scheduling problems on the shop floor are categorized as NP (Nondeterministic Polynomial Time). This classification implies that finding the optimal solution is impossible due to the multitude of variables (lines, work orders, employees, etc.), constraints (work order interdependence, budget, limited resources, etc.), and conflicting objectives (cost minimization vs. due dates of work orders).
While achieving the absolute best solution is impossible, AI models with high computational power excel in finding optimal-like scheduling solutions for manufacturers within the given constraints and objectives. Thus, it can generate significant annual financial returns for large undertakings.
For various manufacturing industries, different application of AI-schedulers can be more profitable such as:
- Changeover Minimization for Pharma: In our OEE benchmarking analysis for pharmaceutical manufacturers, it was revealed that planned downtime constitutes a substantial 31% of staffed time. This figure is notably influenced by stringent quality compliance regulations like cGMP, with their impact reflected in extended changeover periods. For Pharma 4.0 companies, these downtimes can be reduced by 22% by strategically replacing major changeovers with minor changeovers through the implementation of AI schedulers.
- JIT Delivery for Food and Beverage: Food and beverages have a limited shelf life, requiring timely delivery and minimal inventory to enhance profitability. Algorithms and heuristics play a crucial role in minimizing production to meet customer demand and ensure the delivery of fresh products.
- Maximizing On-Time In Full (OTIF) for Consumer Goods: Companies in this industry may face significant financial penalties for late or incomplete deliveries, as they are frequently contractually obligated to meet strict On-Time In Full (OTIF) expectations, often with a target OTIF score. AI algorithms mitigate the risk of incurring OTIF penalties by strategically scheduling work orders based on their proximity to the due dates.
3. Detect Anomalies to Optimize Productivity & Quality
By establishing a baseline of typical behavior, AI can swiftly identify anomalies, be it in production workflows, quality control procedures, or data handling processes. This real-time monitoring capability not only enhances overall security but also ensures the integrity and compliance of manufacturing operations.
For this AI use case in manufacturing, executives can leverage computer vision models to streamline data entry for manufacturing sites. These models interpret stack lights and issue notifications when lines are offline, reducing manual data entry efforts. Over time, these models can collaborate with predictive maintenance models to predict line status by analyzing patterns in green, blue, yellow, and red lights.
Moreover, the integration of diverse data sources, including information on equipment status, environmental heat, electricity currents, and machine vibrations, can significantly elevate production monitoring and predictive capabilities. This comprehensive approach not only enriches the overall data landscape but also enables more effective anomaly detection on the shop floor.
4. Computer Vision Driven Quality Control Inspections
Computer Vision Driven Quality Control (CV-QC) is transforming inspection processes for manufacturers by leveraging advanced algorithms and artificial intelligence to analyze visual data from production lines, ensuring automated quality control inspections.
Traditional quality control methods, reliant on human inspectors, are time-consuming, subjective, and prone to errors. In contrast, CV-QC brings standardization to the process. Cameras and sensors capture real-time images of products, and sophisticated algorithms process this visual data to detect defects, deviations, or potential risks regarding workers’ working condition.
It is crucial to highlight that this AI use case demands a substantial training period. Involving humans in the loop and employing supervised machine learning are often essential to train computer vision models to achieve high accuracy. These training models necessitate constant human feedback until models become proficient in the assigned task. The data labeling process, specifically image labeling, is paramount to teach computer vision models the meaning of visual data. Thus, implementation of computer vision models for quality training can be difficult for many manufacturers.
5. Augment AI and Workers with Digital Twins
Digital twins, also known as AI simulations, serve as valuable tools for manufacturers, enhancing both their AI capabilities, especially in deep learning, and the skills of their workforce. To explain both use cases we will divide section into two pieces:
5.1 Augmented Workers with Digital Twins
Digital twins have the capability to visualize data, enabling executives to gain insights. As illustrated in the image below, for instance, a digital twin can highlight equipment pieces with high degradation using reddish colors, aiding workers in pinpointing the exact maintenance needs of the equipment.
Another use case of digital twins for manufacturers is training their employees through realistic simulations. Similar to flight simulation applications for training pilots, laborers can undergo training to operate equipment, and executives can explore new shop floor organizational methods without any real-world risks.
5.2 Augmented AI with Digital Twins
Deep learning models, exemplified by AlphaGo (an AI system which mastered the ancient game of Go), autonomously refine their performance through iterative training processes. AlphaGo’s mastery of the game through thousands of iterations showcases the capacity of these models to discover optimal strategies, enabling them to surpass human capabilities in specific tasks.
Digital replicas of factories offer a similar playground for deep learning models in manufacturing. For instance, a scheduler module can try numerous scheduling combinations in a digital twin and, like humans, learn by mistakes to make optimal decisions for its speciality.
Digital twins are also essential for master data optimization, a prerequisite for excellent schedule adherence. For instance, if your master data predicts a 30-minute changeover between two work orders, but past data from digital twins shows a 45-minute duration, the AI scheduler uses this empirical information to modify the scheduling. Manufacturers are able to create more effective and realistic schedules as a result.
To learn more about scheduling adherence you can read our In-Depth Guide to Schedule Adherence Report for Manufacturers article.
FAQs
What is AI in manufacturing?
An automation or augmentation tool that helps industrial facilities increase visibility, agility, and productivity. AI excels in addressing intricate challenges that surface regularly on shop floors, such as predictive maintenance and scheduling, due to its ability to handle substantial computational demands.
What AI-related expectations do we have for the manufacturing sector going forward?
As of now, the adoption of AI remains relatively low in the manufacturing industry. Overall, manufacturers are trailing in the integration of new technologies. However, we anticipate a widespread adoption of the technology, particularly in areas like predictive maintenance and scheduling, offering substantial potential for enhancing productivity and profitability.
How Long Does AI Deployment Take for Manufacturers?
This depends on the AI use case you prefer, your capabilities and whether you prefer in-house AI development or outsourcing.
Let us assume you want to deploy an ML model for predictive maintenance. Successfully deploying such a model first requires a robust dataset to identify meaningful correlations among events, including:
- Mean time to failure
- Mean time to repair
- Mean time between failures
- Production speed of machines
- Employee interactions with machines
- Heat and vibration of machine and breakdown and more.
To procure high-quality data for these purposes, the utilization of industrial IoT tools is essential. Additionally, proficiency in algorithm enrichment is paramount. In summary, if your digitalization level is low and lacks skilled IT/developers, the process of designing an AI model may span years, if not decades. In such circumstances, collaboration with a technology vendor becomes imperative to guarantee the attainment of high-quality data and the development of customized algorithms tailored to your shop floor requirements in a short period of time.
How can SCW.AI Support Manufacturers in Implementing AI?
As depicted in the image above, SCW.AI develops specialized algorithms for distinct AI applications in manufacturing. The company also ensures efficient data collection through IoT tools. Consequently, we provide effortlessly scalable cloud-based AI solutions tailored specifically for manufacturers, eliminating the requirement for extra hardware. Guaranteeing both cost-effectiveness and swift deployment, our offerings are designed to streamline processes and enhance operational efficiency. To improve your profitability with AI, you can book a demo with us today!