Artificial intelligence (AI) has emerged as a game-changer for the pharmaceutical industry, offering immense potential to revolutionize operations, from production optimization to research and development. A recent PwC study underscores the promise of AI, projecting that innovative pharmaceutical companies could see their operating margins climb from 20% today to over 40% by 2030 with strategic AI adoption. The study estimates that AI-driven improvements in efficiency and revenue generation could contribute over $250 billion in value within the next five years.
However, realizing this potential requires a clear strategy for AI adoption. Many pharmaceutical companies struggle with identifying and prioritizing high-impact use cases. Notably, AI applications in manufacturing and supply chains, projected to account for 40% of the additional $250 billion, remain underutilized, with only a small fraction of shop floors leveraging AI today.
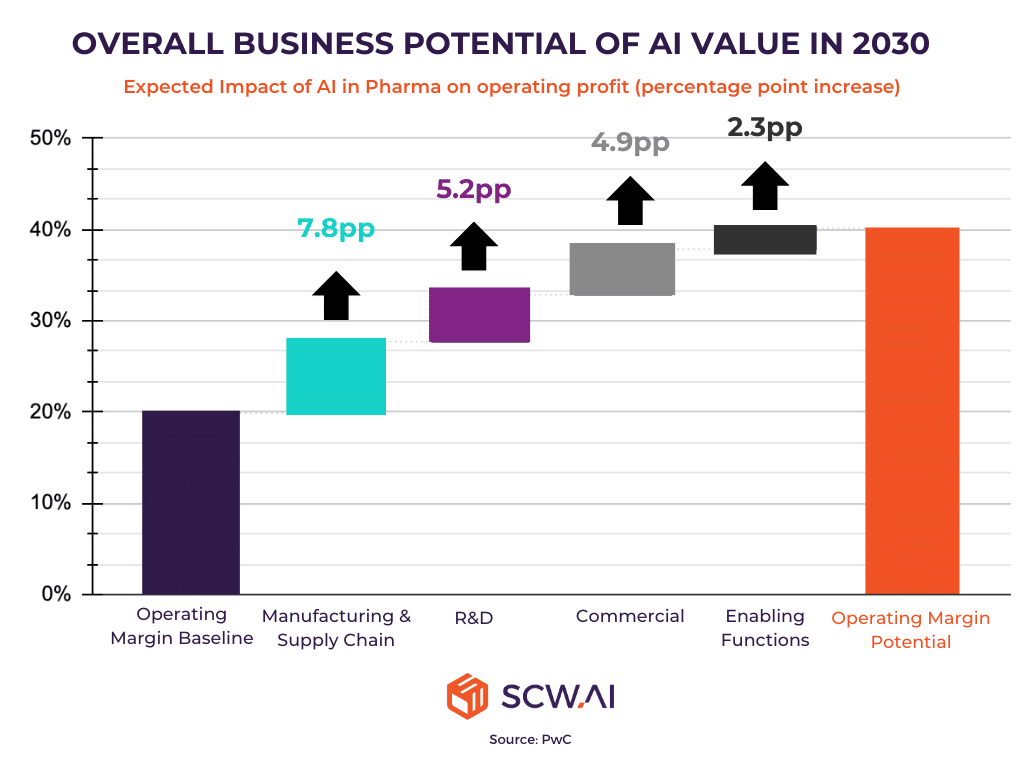
This article aims to provide a roadmap for AI in pharma. We will explore over 10 transformative AI use cases, share inspiring success stories, and discuss the challenges that pharma companies face in adopting AI technologies. We will also look ahead to the future of AI in pharma and introduce our AI HUB, a solution designed to help pharma companies embark on their AI journey. Finally, we have included a comprehensive (expanded) downloadable PDF version of this article to serve as a handy resource for pharma professionals navigating the evolving AI landscape.
What is AI in Pharma?
Artificial intelligence (AI) is an intelligent automation solution designed to perform or assist with tasks traditionally carried out by humans, such as pattern recognition, problem-solving, and decision-making. In the context of the pharmaceutical industry, AI offers transformative capabilities across various domains (we will see in detail later), including manufacturing, R&D and more.
For instance, in pharma manufacturing, AI can optimize scheduling and enable predictive maintenance to enhance operational efficiency. In R&D, it can assist with optimal patient recruitment for clinical trials or interpret vast datasets, such as DNA sequences, to accelerate drug discovery. These applications highlight the versatility of AI in addressing both operational and scientific challenges.
At its core, AI relies on algorithms to train models that complete tasks, which can broadly be categorized into supervised learning and unsupervised learning:
- Supervised Learning: In this approach, AI systems are trained on labeled datasets, where each data point is associated with a known output (e.g., images labeled as “cat” or “dog”). The algorithm learns to map inputs to outputs by minimizing the difference between its predictions and the actual labels. This method is widely used for tasks like classification (e.g., anomaly detection) and regression (e.g., predictive maintenance, demand prediction).
- Unsupervised Learning: Unlike supervised learning, this approach works with unlabeled data, where the goal is to identify patterns, structures, or relationships without any predefined output labels. Common tasks include clustering (AI drug discovery) and dimensionality reduction (e.g., simplifying large datasets while keeping essential information). Unsupervised learning is mostly used for exploratory data analysis.
Both approaches have distinct advantages and limitations. Supervised learning provides more controlled and predictable results, whereas unsupervised learning offers scalability and adaptability, particularly for analyzing complex and unstructured data.
On the other hand, the success of any AI algorithm hinges on two critical factors which require a perfect digital infrastructure:
- Quality of Data: Accurate, relevant and comprehensive data ensure robust and reliable outputs.
- Computational Power: The availability of scalable computing infrastructure is essential to support AI’s full potential in pharma applications.
In the episode 5 of SCW for Pharma Podcast series, Mike Walker, Microsoft’s Executive Director of Global Healthcare and Life Sciences Digital Strategy, explains the importance of having a robust digital ecosystem for utilizing AI in pharma effectively.
10+ AI Applications in Pharma
In this section, we will explore top AI applications in pharma, which are expected to generate approximately $250 billion in value by 2030.
1. Job Shop Scheduling
AI-driven production scheduling is one of the most impactful applications of AI in pharma manufacturing. According to PwC, augmented scheduling solutions are expected to generate $12–25 billion in value by 2030. These systems can reduce operational costs by up to 10% while significantly improving throughput, depending on the chosen optimization scenarios.
The complexity of job shop scheduling makes it a perfect candidate for AI intervention. Scheduling involves managing hundreds of work orders, multiple production lines, and numerous stations with intricate interdependencies. Constraints—both hard (e.g., compliance with cGMP and quality standards) and soft (e.g., cost efficiency and customer satisfaction)—make scheduling problems notoriously challenging, often categorized as NP-hard problems. In such cases, reaching a truly optimal solution is virtually impossible. Instead, AI leverages heuristics, algorithms, and computational power to deliver near-optimal results more efficiently.
How AI Enhances Job Shop Scheduling
AI solutions excel in orchestrating complex scheduling tasks with greater precision and speed. They can create optimized schedules in 50% less time than traditional methods. These systems are trained to address specific business scenarios, including:
- Minimizing changeovers.
- Enabling just-in-time production.
- Maximizing on-time, in-full (OTIF) delivery performance.
- Reducing overall costs and many more.
Once trained with historical data, AI models can generate tailored schedules with just a click (See Video Below), offering unparalleled agility and accuracy.
AI-Powered Simulations for Enhanced Decision-Making
AI-powered scheduling simulations enhance the capabilities of scheduling staff and foster collaboration between humans and AI. For example, in the case below, we ran 88 work orders using different algorithms, each with unique expectations for OEE, capacity utilization, cost, and other manufacturing KPIs. Experienced schedulers can choose the optimization scenario that best aligns with their business needs and, if necessary, refine it further manually. This approach enables schedules to be more responsive and agile, going beyond mere cost minimization.
2. Predictive Maintenance
Predictive maintenance is a high-value AI use case in pharma, projected to generate around $10 billion by 2030 due to cost savings from reduced unplanned downtime. AI algorithms analyze a variety of inputs, including machine vibration, heat, sound, electrical current, and statistical data like mean time to failure (MTTF) and mean time to repair (MTTR).
By processing these inputs, AI can predict machine failures before they occur, enabling maintenance to be scheduled proactively. To optimize costs, the algorithms aim to minimize unplanned downtime while keeping planned maintenance durations as short as possible. Thus, predictive maintenance offers a digital lean and total productive maintenance solution for the pharma manufacturers.
3. Computer Vision-Driven Quality Checks
Computer vision, the technology enabling autonomous vehicles to sense their environment, is a powerful AI application in pharma. Given the industry’s stringent cGMP requirements and world-class manufacturing standards, ensuring consistent quality is paramount.
AI-powered computer vision systems can identify scraps and units requiring rework in real-time, enabling immediate corrective actions. This not only boosts labor productivity—allowing staff to focus on value-added tasks—but also helps monitor and improve first-pass yield over time. The result is higher product quality and reduced costs through fewer defects and more efficient processes.
4. Anomaly Detection and Digital Twin of the Factory
Anomaly detection combined with digital twins is a cutting-edge AI use case in pharma, enabling manufacturers to identify and replicate the “golden batch” that minimizes deviations and rework.
Digital twins leverage historical factory data, including machine configurations, operator assignments, environmental conditions (e.g., humidity and temperature), and more. By correlating these input variables with output indicators (KPIs) like OEE, cost of quality, schedule adherence, etc. AI algorithms uncover optimal settings for production. Simulations then allow the factory to determine unique machine speeds and worker allocations for each line, ensuring minimal deviation from the golden batch.
Once the golden batch is identified, anomaly detection systems monitor for deviations, triggering alerts when discrepancies occur—such as a machine operating at an incorrect speed—so corrective actions can be taken promptly. This capability ensures consistent batch quality.
The impact on cost and quality can be significant. A McKinsey study found that top-performing pharma manufacturers experience 14 times lower quality costs compared to their peers, thanks to achieving just 1/6th of the deviations per 1,000 batches of their average competitors.
5. Automated Action Assignment
Pharma companies can leverage AI to automate repetitive task allocations, freeing managers to focus on strategic priorities. AI-driven management tools, such as Action Trackers, analyze data over time to identify patterns—like assigning maintenance teams during unplanned downtimes or cleaning wet floors to prevent accidents.
Once these task allocation rules are established, AI automatically assigns actions, ensuring efficiency and standardization while reducing the burden of routine decision-making on managers.
6. Demand Forecasting and Inventory Management
AI is transforming demand forecasting and inventory management in pharma by providing more accurate predictions and enabling data-driven decision-making. By analyzing historical sales, market trends, seasonality, and external factors, AI algorithms can forecast demand with higher precision, reducing stockouts and overstocking. This improved accuracy allows pharma companies to align production schedules, reduce costs, and make better strategic moves.
As discussed in Episode 6 of the SCW for Pharma podcast, Tolga Kula, Director and Global Head of Integrated Business Planning at Merck Group, highlighted how AI-augmented demand forecasting enhances precision and empowers organizations to stay competitive.
7. Smart Logistics
AI also improves supply chain logistics in pharma by optimizing routes to reduce costs, delivery times, and carbon emissions. With AI-powered route planning, companies can streamline the transportation of raw materials, intermediates, and finished products. This ensures faster deliveries, lowers expenses, and supports sustainability goals—all critical in today’s competitive pharma landscape
8. AI in Pharma Sustainability
According to the World Economic Forum, pharma companies emit 1.5 times more greenhouse gases than the automotive sector per $1 million in revenue. By integrating AI into sustainability strategies, pharma companies can reduce their carbon footprint per dollar in following ways:
- Carbon Emission Simulations: AI-driven carbon trackers analyze factory activities to identify the main sources of greenhouse gas emissions. Much like finding the golden batch, these tools pinpoint bottlenecks and propose actionable steps to reduce pollution.
- AI Scheduling: For factories using solar panels, wind turbines, or energy from green producers, AI can optimize energy-intensive activities by aligning them with peak sustainable energy availability, considering factors like weather and daylight.
9. AI in Clinical Trials
Clinical trials are a critical phase in drug discovery, ensuring medicines are both effective and safe. AI accelerates clinical trials, reducing time to market for life-saving treatments. The technology enhances this process in three key ways:
- Recruitment for Testing: AI identifies and matches suitable participants for trials based on medical history, demographics, and eligibility criteria, speeding up the recruitment process.
- Monitoring Patient Data: AI continuously analyzes patient data during trials to detect adverse reactions early, improving safety and compliance.
- Analyzing Trial Data: AI uncovers complex relationships within trial data that are difficult for humans to detect, offering deeper insights into drug efficacy and potential new applications.
10. AI in Drug Discovery
AI speeds up the drug discovery process by analyzing how molecules and biologics interact with diseases, paving the way for advanced therapeutic solutions. It plays a crucial role in protein folding and protein backbone torsion angle prediction—key factors for developing Advanced Therapy Medicinal Products (ATMPs). AI also predicts the potential efficacy of drugs by examining historical clinical trial data, significantly accelerating the identification of promising candidates.
For a deeper understanding, watch the inspiring lecture by 2013 Nobel Laureate Dr. Michael Levitt on AI-powered drug discovery, which highlights the immense potential of AI in revolutionizing this field.
11. AI in Drug Repurposing
AI is proving valuable in drug repurposing by identifying new therapeutic uses for existing drugs. By analyzing vast datasets, including clinical trial results, chemical structures, and biological pathways, AI can uncover hidden relationships between drugs and diseases. This approach helps pharma companies better manage their risks through diversification and economies of scope.
12. AI in Pharmacovigilance
In pharmacovigilance, AI helps monitor and analyze data from adverse event reports, clinical trials, social media comments, and real-world evidence to detect safety concerns more efficiently. It can identify patterns and trends in large datasets, enabling faster detection of potential risks.
13. Generative AI in Pharma
Generative AI is a versatile tool capable of replicating many AI applications discussed, such as generating digital twins or optimizing job shop scheduling, when properly trained. Beyond these, it supports marketing, sales, finance, and IT teams in creating reports, writing code, or crafting outreach emails. Therefore, a McKinsey study estimated that within a few years generative AI can create $60 billion to $110 billion additional value for the pharmaceutical and life sciences industry.
Yet, its true value lies in its creative potential. As the pharma industry undergoes rapid transformation—driven by emerging technologies, regulations, and innovations like ATMPs, biologics, and orphan drugs—upskilling workers becomes essential. Generative AI can act as an effective teacher by creating personalized training videos, interactive quizzes to assess learning, and real-time support for tasks like protein torsion angle prediction to compliance with new manufacturing standards and many more.
5 Inspiring AI Case Studies for Pharma
1. Johnson & Johnson India: Predictive Maintenance and Demand Forecasting
Johnson & Johnson’s Mulund factory in India, part of the WEF Global Lighthouse Network (less than 2% of factories worldwide as of 2024), achieved transformative results with AI/ML. Predictive maintenance reduced unplanned downtime by 50%, while smart demand forecasting and inventory management improved OTIF scores by 4.5 percentage points.
2. Cipla India: Reduced Changeover Duration by 22% with AI Scheduling
Cipla’s Indore facility, also a Lighthouse member, reduced changeover durations by 22% through AI-driven job shop scheduling. Full supply chain visibility and intelligent automation further cut manufacturing costs by 26%.
3. Agilent Technologies Singapore: Improved Labor Productivity by 31% with AI Inspections
At Agilent Technologies’ Singapore site, computer vision-driven quality checks increased labor productivity by 31%, allowing staff to focus on value-added tasks. Additionally, digital twin simulations helped identify golden batches, reducing manufacturing costs by 25%.
4. Insilico Medicine: Reached Phase 1 in 50% Less Time
Source: Science Direct
5. FDA's Sentinel Initiative Program
The FDA’s Sentinel Initiative uses AI to monitor real-world data for adverse drug events and safety signals, improving pharmacovigilance and enhancing patient safety on a large scale.
Top 5 Challenges of AI in Pharma
1. Data Privacy, Security, Quality, and Availability Concerns
- GDPR and HIPAA Compliance: Ensuring compliance with data protection regulations is a significant hurdle.
- Data Breaches: Over one-third of breaches now cost over $1 million, according to PwC. Thus, lack of cybersecurity infrastructure can lead to less ROI by AI.
- Digital Ecosystem: AI relies on accurate and rich data, which requires robust digital infrastructure. Automating data collection is essential to ensure data quality, accuracy and scalability. Thus, to build a predictive maintenance model for instance, pharma manufacturers should have solutions like OPC connections, IoT sensors, and manufacturing analytics.
2. Integration and Change Management
Successfully implementing AI requires seamless integration with existing systems and a culture ready for digital transformation. Resistance to change, lack of alignment between departments, and outdated systems can slow progress. Effective change management strategies are crucial for overcoming these barriers.
3. Skilled Labor Shortage
The demand for skilled professionals to design, deploy, maintain and operate AI systems often exceeds supply. For instance, in Germany, 30% of IT-related positions in the pharmaceutical sector remain unfilled, highlighting the industry’s struggle to attract and retain talent.
4. Regulatory Compliance
Navigating the complex regulatory landscape poses a significant challenge for AI in pharma. Ensuring that AI tools and processes meet stringent legal and ethical standards requires continuous monitoring and adaptation.
5. Time to Value and Future Legacy Costs
Pharma companies face pressure to achieve fast ROI from AI investments. Collaborating with cloud providers often ensures quicker implementation compared to in-house AI development per PwC. On the other hand, the rapid pace of technological evolution, as described by Moore’s Law, means companies must consistently upgrade AI tools or partner with technology vendors to avoid obsolescence.
Future of AI in Pharma
SCW.AI anticipates that AI models will become increasingly effective, driving cost reductions and revenue growth across the pharmaceutical sector. However, the ROI and time to value will depend on how companies address challenges and prioritize AI investments, as well as national policies that support AI adoption.
The benefits of AI adoption will not be evenly distributed. Companies that act decisively will gain a competitive edge in several areas:
- Cost Efficiency
- Quality
- Innovation
- Sustainability
- Sales
- Attracting Top Talent
Slower adopters, on the other hand, risk falling behind. This makes it essential for the Department for Digital Excellence and top management to lead efforts in change management, preparing digital infrastructure, and selecting the right technology vendors.
The pharmaceutical industry’s strategic importance also plays a significant role. The COVID-19 pandemic and disruptions in globalization, such as trade wars, have underscored this point. Regions like the European Union, which are expected to generate only one-fifth of the AI value compared to U.S. companies may face greater productivity gaps. These disparities could negatively affect drug availability and the effectiveness of medicines in the coming decades.
If you are a European pharmaceutical manufacturer and want to learn the reasons for the productivity gap with the U.S. and China, as well as discover practical pharma 4.0 tips to minimize it, download our eBook: “The European Pharma Productivity Gap Report: From Policy to Practice“
About SCW.AI’s AI HUB
SCW.AI is dedicated to enhancing drug availability and quality worldwide by empowering life sciences manufacturers with turnkey Pharma 4.0 solutions through our comprehensive Digital Factory Platform. With over a decade of experience in digitizing pharmaceutical manufacturers, we pride ourselves on delivering simple, scalable, and rapidly deployable solutions tailored to the industry’s unique needs.
AI HUB is our intelligent automation solution designed specifically for manufacturers, offering:
- AI-driven scheduling and simulation generation.
- Anomaly detection.
- Automated task assignment.
- Custom AI algorithms for predictive maintenance and digital twins.
If you have questions about AI in pharma or our Digital Factory Platform, contact us today.
To experience our cutting-edge innovations and see the future of pharma manufacturing in action, book a demo now.